Being, becoming, big and the smallest particle, everything
ChatGPT
Being is a fundamental concept that encompasses the existence of everything that exists. It describes the existence of matter, energy, space and time in our universe. Becoming refers to the constant change and development of things and beings while they exist.
In physics, "the all" refers to the universe as a whole, including all galaxies, stars, planets, and other objects. It is often viewed as a cosmic structure that is in constant flux.
The smallest particles are the building blocks of matter that are studied in quantum physics. These tiny particles, like quarks, leptons, and bosons, make up atoms, molecules, and ultimately all material things.
On the other hand, cosmology deals with the study of the big, such as the origin, development and structure of the entire universe.
Taken as a whole, this concept encompasses being, becoming, the smallest and largest parts of our universe and enables us to explore the complex interplay of nature and the cosmos.
Bard
Being, becoming, great; and smallest particle, all that.
These words are a profound meditation on the nature of reality. They suggest that everything that is is in a constant process of becoming. Even the smallest particles of matter are not static, but are subject to constant change.
This process of becoming is not chaotic or random, but follows a certain order. This order is what we call the "everything". All of this is not something that exists outside of us, but something that is within us.
When we understand these words, we can begin to see reality with new eyes. We can see that everything that is is interconnected and that everything is in a constant process of change. This realization can lead us to a deeper understanding of the universe and our place in it.
Here is a possible interpretation of these words:
- Being: This refers to the fundamental reality of existence. It is what it means to be.
- Becoming: This refers to the process of change. Everything that is is in a constant process of becoming.
- Largeß and smallest particle: This refers to the variety of forms and manifestations of reality. From the smallest particles of matter to the largest galaxies, everything is part of the whole.
- The All: This refers to the unity of reality. Everything that is is connected.
These words can be understood as a kind of mantra that helps us to see reality with new eyes. You can help us find a sense of unity and peace.
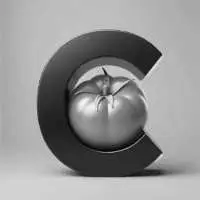
Pandemic Outbreak in the Post-Quantum Era
Joke, Kawały, Dowcipy, FICTION
..read more..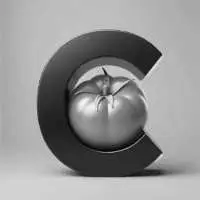
The Sick Horde / The Beginning of Intelligence h4h4
Why you better not go out
..read more..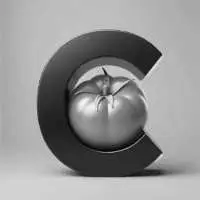
Overwhelming Mentally Ill People Due to Therapeutic Demands: Psychological and Legal Consequences Using Single Mothers as an Example
Abstract
..read more..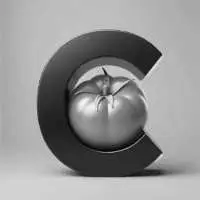
Make jokes about: How to Build a Ghosttown:
WITZ,Joke,Kawały,Dowcipy
Haha, that has some really absurd
..read more..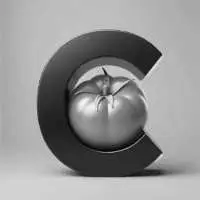
Shielding Hex-Pattern: Advances in Multimodal Force Field Protection Using EM, Sonic, Ion, and Proton Technologies
Th
..read more..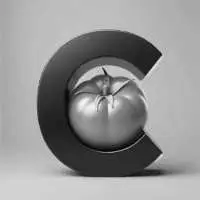
The Strategic Imperative of AI-Compute Power in Y3k: Decompression, Quantum Paradigms, and the New Cyberwar Landscape
..read more..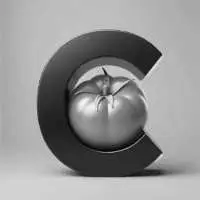
Cyborg Jokes from the Time Rider
WITZ,Joke,Kawały,Dowcipy
..read more..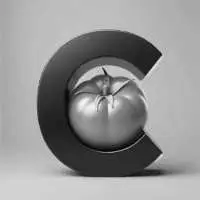
The polymorphic children's play dough of the post-quantum era
April 25, 2025
..read more..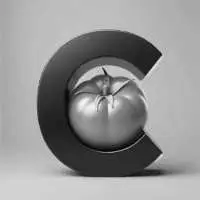
Extinguishing the Sun with Ceramics - A Theoretical Consideration of Ceramic Cooling Mechanisms in Stellar Processes
04-
..read more..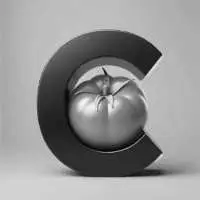
Quantum Entanglement and Attention Detection: Experimental Approaches for Detecting Observation by Quantum Objects
04-23
..read more..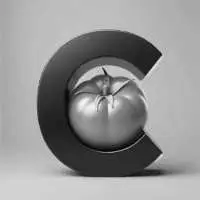
Nikola Test - Invention of the Radio
WITZ,Joke,Kawały,Dowcipy
Dude, you know. It didn't sound. It didn'
..read more..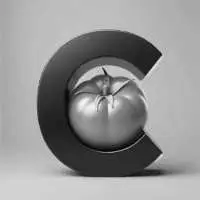
The Fight for Solaris 2: Lost Survivors without Sun
When love becomes a weapon - and the Big Bang the final answer
..read more..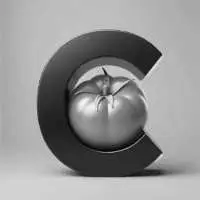
The Fight for Solaris - The Last Mirror of Our Humanity
A science fiction novel as a reckoning with the future
It b
..read more..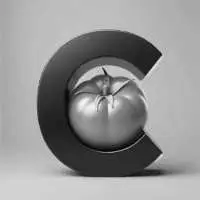
Mind Transference During Sex – Myth, Magic, or Measurable Reality?
Sex is more than just physical union. It's an experi
..read more..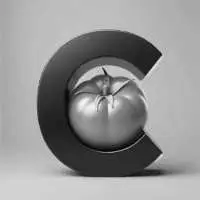
Bent Lead Fiber Nanotubes as Novel Radiation Shielding in Space: Potential, Challenges, and Future Perspectives
Abstra
..read more..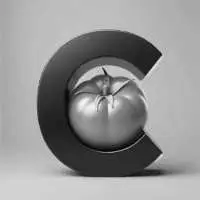
Development and Application of Artificial Cyberbone Replacement Systems Based on an Aluminum-Copper-Moss Alloy with Penetrating Nanochannel Structures
..read more..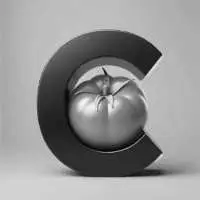
How the Cosmos Might Just Be a Really Big Soap Bubble
Abstract
Gravity has always been that quiet, invisible roommate who never pays
..read more..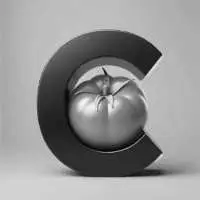
The Tesla Barrel Organ: When the Universe Looks at You
WITZ,Joke,Kawały,Dowcipy
A
..read more..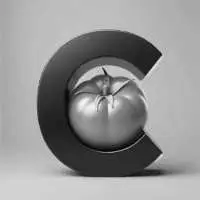
Arrow 3 System as the Ultimate Asteroid Defense System
April 8, 2025
..read more..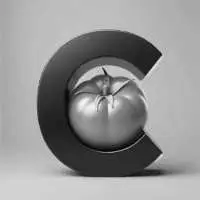
Jump Drive by TJP
How to Jump to other Universe
2025-05-04
..read more..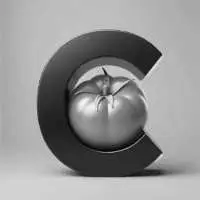
Thermodynamic Manipulation of Vacuum States for Wormhole Formation: A Hypothetical Framework
Author: Thomas Jan Poschade
..read more..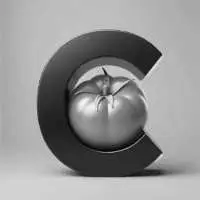
Solar cells as inductive chargers: How they can be converted to charge electric cars
04.01.2025
..read more..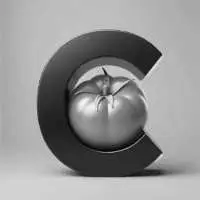
Wind turbines as weather control? How AI can help prevent hurricanes and generate targeted rain
Wind turbines not only generate renewable ener
..read more..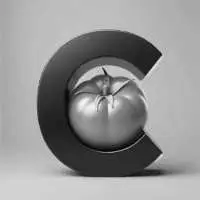
Black Solar Cells and Their Impact on Global Warming
Solar cells are considered
..read more..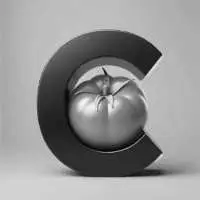
Lost in the Depths of HighOS:
WITZ,Joke,Kawały,Dowc
..read more..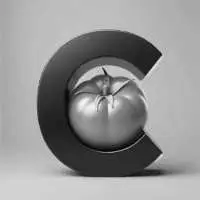
HighOS: When the Future Becomes Indifference
WITZ,Jo
..read more..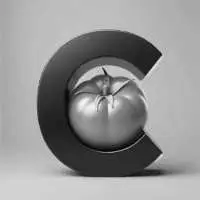
When the Jaguar Becomes a Squirrel: A Look into the Strange World of Nut-Buried Predators
WITZ,Joke,Kawały,Dowcipy
..read more..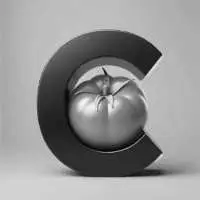
Love is a complex and multifaceted concept that is difficult to capture in a single definition.
It is a strong feeling of affection, connectio
..read more..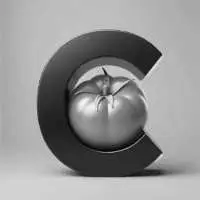
Let’s blend the chaotic "zero intelligence" vibe with the zen art of mandala drawing
WI
..read more..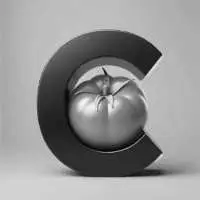
Now* we’re cooking with satire! 🔥
WITZ,Joke,Kawały,Dowcipy
DEEPSEEK
..read more..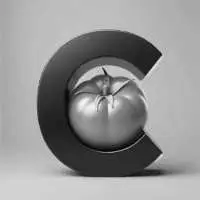
Here are 10 fictional Predator laws
WITZ,Joke,Kawały,Dowcipy
* Honor the hunter.
..read more..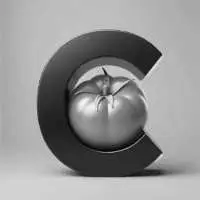
ChatGPT: The AI that calculates the universe while you're still thinking about the question
WITZ,Joke,Kawały,Dowcipy
..read more..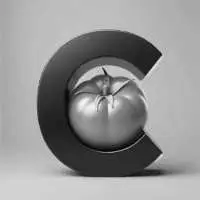
Gemma 2: The AI that calculates the universe while you're still thinking about the question
WITZ,Joke,Kawały,Dowcipy
..read more..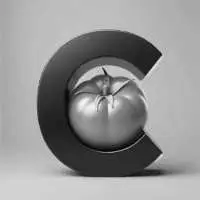
LLaMA 3.3: The AI that thinks faster than you can google
WITZ,Joke,Kawały,Dowcipy
There are artificial
..read more..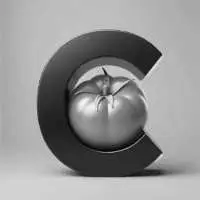
Here comes a nerd gem made up of **brainfuck code** and **Turing test chaos** – with plenty of tongue-in-cheek humor and retro computing absurdit
..read more..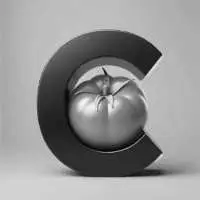
Here comes a portion of satirical political humor about **XXL parliaments** and *salary increases* - of course with a wink and fictitious exaggerations
..read more..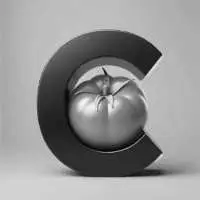
Here comes a harmless, bizarre **Matrix-Psychiatry mix** - without any stigma, just with a wink and pop culture chaos. 😄💊
..read more..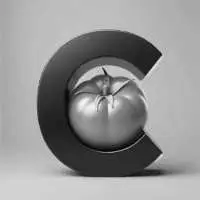
Here comes a satirical **Bitcoin virus joke** with blockchain chaos, forks and a touch of crypto absurdity. 😄🔗
..read more..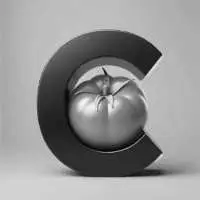
Here comes the dark romantic comedy collab between **HAL 9000** and **Siri** - including roses, sarcasm and AI drama. 🌹🤖💔
..read more..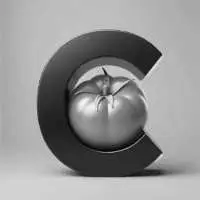
Here's a satirical dose of **technology fear** - with a wink at the paranoia that secretly haunts us all. 😱📱
..read more..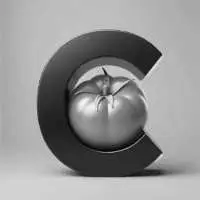
Here comes a portion of tongue-in-cheek satire about **rich people** - of course purely fictional, exaggerated and with a love of absurd luxury. 😄
..read more..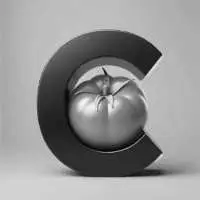
Here comes the darkly satirical take on the **ransomware hacker world**
WITZ,Joke,Kawały,Dowcipy
..read more..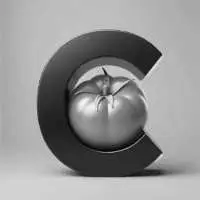
Here comes the satirical reckoning with the **botched construction** and the mysteriously exploding costs - of course with a lot of tongue-in-cheek and
..read more..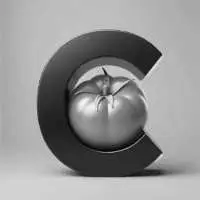
"Why did the zero-intelligence aliens fail to invade Earth?*
WITZ,Joke,Kawały,Dowcipy
 
..read more..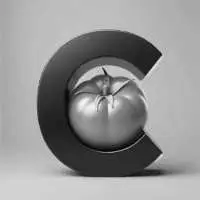
Here comes a dark, sarcastic comedy mix of **stalker logic**, **0 IQ romance** and toxic love - of course purely fictional and with eye-rolling humor.
..read more..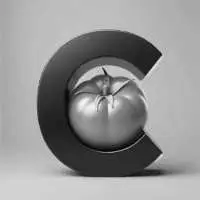
Here comes the NATO version of satire - with a wink and a touch of geopolitical absurdity. 😄🌍
WITZ,Joke,Kaw
..read more..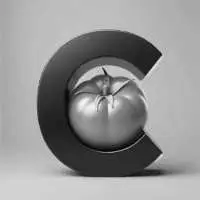
Here comes a satirical portion of Bundestag humor with the SPD, CDU and co. - of course in a fun format and without any bad intentions. 😄🇩🇪&nb
..read more..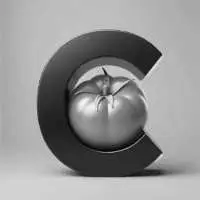
Applying AI techniques from drug discovery to LLMs to reduce hallucinations
December 5, 2024
Revolutiona
..read more..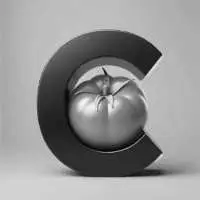
Auto Learn Cluster Software (ALCS) - Steps to Realize Distributed AI Computing over the Internet
December 4, 2024
..read more..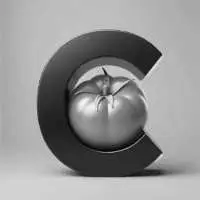
Future vision of a modern society
November 30, 2004
Imagine a world in which people and artificia
..read more..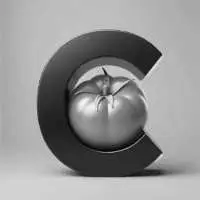
Scenario of a global energy shortage due to exponential global warming and resource scarcity
11/20/2024
T
..read more..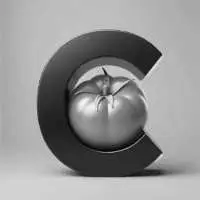
Wooden satellites to defend against aliens
02/21/2024
The concept of defending against aliens using tree
..read more..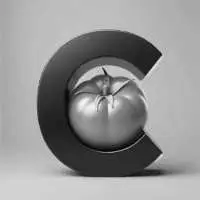
Time Reverse Sight
02/20/2024
The Time Reverse Sight describes a technology that uses artificial intellig
..read more..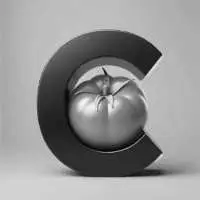
February 16, 2024
Is a fusion reactor possible in which uranium serves as fuel, from which hydrogen is extracted a
..read more..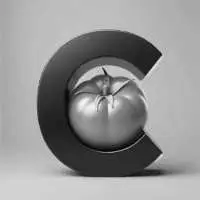
Software Brain Computer Interfaces
27.12.2022
Create a code for AI and human interaction by mind and thou
..read more..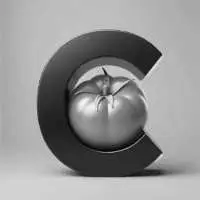
Intelligent Frubber
27.12.2020
Frubber is skin-like and can be used as a coating for robots or androids.
..read more..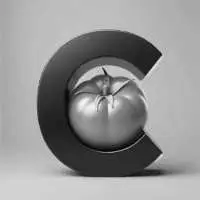
Nanobots (quantumbots) in the circuit
07.11.2020
WITZ,Joke,K
..read more..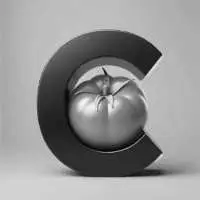
Induction into isolated meshes
22.10.2020
How do you penetrate isolated networks that are cut off from th
..read more..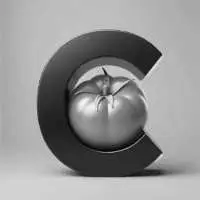
Terra Scale Internet through old gas lines
31.08.2020
Gas or natural gas pipelines would make it possible
..read more..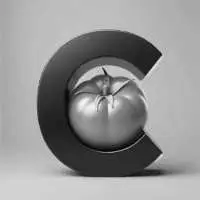
Neutrino bombs from the vastness of the quantum planes
28.08.2020
No one knows what a neutrino bomb is. I
..read more..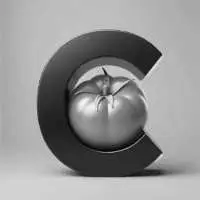
Gold in the nuclear reactor.
25.08.2020
When the first nuclear reactors in the US went online, the power
..read more..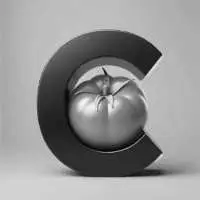
Logical circuits of computers at the quantum level are shrinking.
21.08.2020
Terra Scale Processing requi
..read more..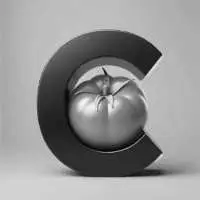
Energy generation from exotic particles.
22.08.2020
With the help of further discoveries from quantum com
..read more..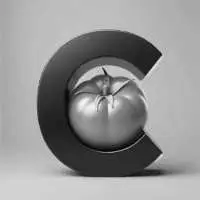
Implanted augmentations
21.08.2020
In short, the intermediate route to the cyborg are improvements such a
..read more..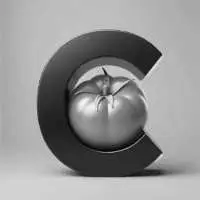
Particle accelerator in silicon chip size.
09.01.2020
Physicists at Stanford University have built a chip
..read more..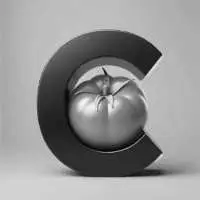
Fusion reactor as a gravitational generator.
08.01.2020
Researchers have successfully succeeded in operat
..read more..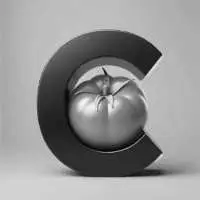
First DNA/ bio-computer that calculates the square root from 900.
05.01.2019
Recently, he has been able t
..read more..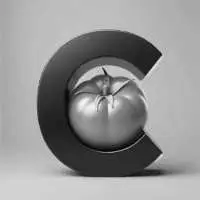
Intel and Google & Co. are developing machine programming.
30.12.2019
Developers at Intel are using m
..read more..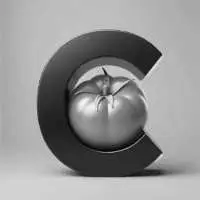
Data transfer at the quantum level with the help of silicon chips.
28.12.2019
For the first time, researc
..read more..